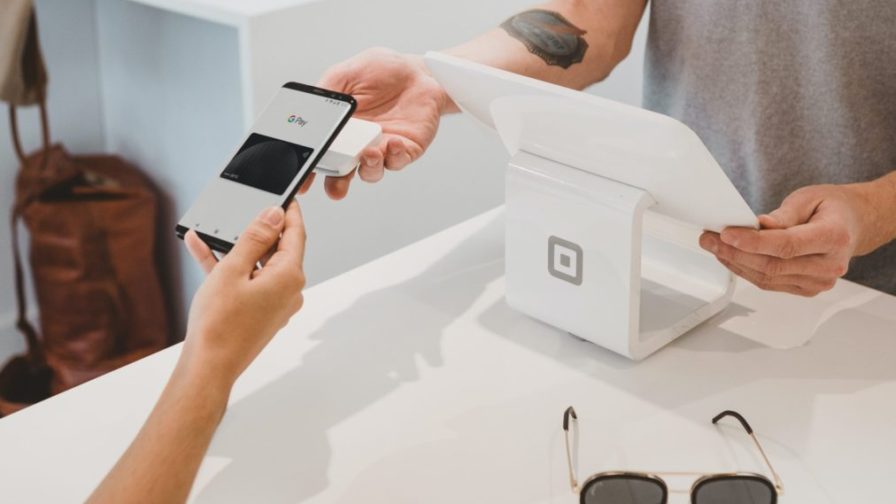
In preparation for Codemotion Deep Learning conference, we had spoken to one of the speakers, Manuel Morales. He wears a variety of hats in machine learning. Since 2018, he has been General Director of the FinML Network.
He is currently an Associate Professor in the Department of Mathematics and Statistics at the University of Montreal specialising in Financial and Actuarial Mathematics, specifically the application of Machine Learning in banking and in responsible investment.
He recently participated in the Artificial Intelligence transformation initiative of the National Bank of Canada. As the Chief AI Scientist, he led the scientific efforts of the bank’s strategy to leverage AI technologies across all verticals. He had the opportunity to work on a wide variety of projects from wealth management to retail banking applications, and also led the first works towards laying down an AI governance framework.
He’s also working within the startup ecosystem coming out of the University of Montreal, he noted ” There are many students with the initiative to start companies using technology, machine learning technology and data-driven technology. So I found startups and work within the industry helping other companies to adopt AI technology.”
The technology of personalisation contributes to digital transformation in banking
The convergence of a range of technologies including machine learning and AI have led to the digital transformation of numerous traditional sectors, including banking.
Manuel asserts that we’ve witnessed “fintechs trying to innovate and moving faster than traditional banking institutions” through blockchain technology, AI, and increased computing power.
Part of the impetus if customers who are accustomed to the personalized service of companies like Amazon, Facebook, and Google and subsequently banking became very aware fast of what this technology can can can do for them. So all of them then created pressure for financial institutions to innovate.”
The National Bank of Canada started by putting up the necessary infrastructure. Then they started putting together teams so that we could leverage that data. In terms of the variety of banking verticals Manuel shares that “retail is usually where we create significant impact.
Anything that has to do with our client-facing processes, client onboarding, leveraging our digital channels, where clients interact. But it also applies expansively such as wealth management. How do we use AI to better produce revenue and reduce costs? It spreads through document processing in Human Resources, our legal teams, our accounting teams, operations and marketing.”
AI is more than just statistics and analytics automation
According to Manuel when people started talking about AI in banking, they had this idea that it was just one more level of complexity in terms of models.
“They thought all that all you needed was to hire PhDs in machine learning and then all of a sudden everything would start working the magic. However, it’s not just more sophisticated statistics or models or mathematics. It’s actually an opportunity to, to change the way the processes themselves are designed within.”
Manuel attributes the slower innovation in banking compared to some sectors in part to the need for a cultural shift.
“It’s the mindset that has to change as well because banks are supposed to be digital-born companies. They have a different way of thinking. So what most banks are trying to do now is every time that there’s a deployment of a new application or a new service to clients. there must also be data collection.
When you think about what Facebook and Google are doing, they’re providing services but also a means of collecting more data that allows them to open new markets, identify new opportunities, and better serve the clients. So that’s what we’re trying to do. And that’s the mind shift that has to be pushed within banks.”
The threat and opportunity of fintech startups
In the UK and Europe Fintechs such as N26 and Monzo have provided an alternative to traditional banking services – they use chatbots, offer bilingual services and digital-first banking, unlike classic banking which is heavy of paperwork and still use fax machines.
Manuel notes “Classic banks haven’t been able to move fast enough as younger companies who are taking parts of their market. But I think that changes a lot from country to country. In Canada, it’s not that much of a threat yet, where new entrants are only capturing 2% or 3% of new business, whereas in places like Germany and the UK it’s something like the 20%”.
Yet “there’s the sense of fintech banking as a threat but there’s also a strong sense that banks have the benefit of a strong foundational structure a. Fintechs cannot do many of the things that a bank can. Because it’s a very regulated sector.”
What kinds of tools are used in machine learning in banking?
A range of tools and technologies are in use especially “anything that could improve current processes and help us pass or bypass bottlenecks. So for instance, anything that has to do with natural language processing and document processing and information extraction technologies that’s heavily in use right now. It has a lot of impact because banking involves so many processes especially when you’re trying to onboard clients.
So anything that can reduce those bottlenecks – technologies related to text and document extraction”
Also in use is anything to facilitate knowledge extraction: “Because the idea is building a personalized client profile, as achieved by Google and Facebook. So, they have a profile with thousands of data points for each individual that uses the platform. And even for those that don’t use it, they also have a profile for them.
So the idea is, we have traditional data like transactions: How do you use your card, your bank, or your shop? What kind of shopping do you do? That gives a profile of the client but also, any single interaction that the client has with digital channels like through mobile applications, then you have access to how the client. How do they move around? Where do they go? How are they interacting with the application?
Manuel calls this a ‘carrot and stick’ approach. “Previously if a bank wanted to undertake advanced analytics it would be like I have a lot of data I can put into a model which gives an output.
That output is consumed by a team of people that understand that output and then can make business decisions. It could be a marketing team that then understands better how clients are shopping. So then they would design a marketing campaign around that.”
Now you have data loss models and models give an output which can actually include making business decisions by the model themselves. “And those business decisions have an impact on clients, and then the clients feeds their response back into the data and then there’s a loop there.”
Manuel gave the example of a mortgage application “The customer has usually been shopping around on their phone. So there’s
“What kind of interaction he has with the application? Let’s say he’s making an application for a mortgage. Before doing that he’s been shopping around usually on his phone. There are so many ways you can have extra data points about a client.
But then the challenge is how do you translate that? Or how do you map that into a piece of data information that you can feed into a model? So that’s recommendation systems. Deep Learning for knowledge extraction of knowledge representation.”
You have to think about carrot applications that provide services or ways for clients to interact with digital channels. They provide a service, a better experience for the clients but at the same time, collect data that enhances the capabilities of the bank to provide better services and anticipate new needs and new demands.
Attract clients, so they provide more information about themselves.
If you want to know more about how modern technologies and tools can support you for – and during – the organisation of a virtual event, don’t miss this article showcasing the best tools we used to host our online conferences since the COVID-19 outbreak.