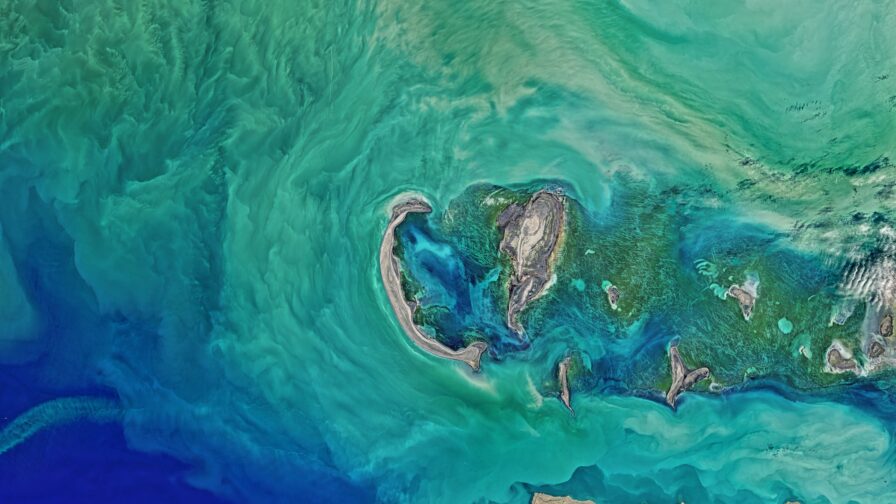
What is Google Earth Engine?
Earth Engine is Google’s cloud-based platform for doing planetary-scale analysis and visualization of Earth Data. It allows its users to access large amounts of geospatial data, such as satellite imagery, while at the same time providing computation power to perform advanced data processing effectively at scale.
Earth Engine is accessible through a website and through a set of public APIs, which enables users to easily perform heavy processing and analytic tasks.
Data
This platform contains a large amount of remote sensing data, more than 700 public datasets. Every month more than one petabyte of data is added to the platform. It currently contains more than 70 PB of data, allowing the monitoring of the Earth’s surface up to 50 years back in time
The data catalog houses a large repository of publicly available geospatial datasets. It includes observations from satellite and aerial imaging systems both in optical and non-optical wavelengths, weather and climate forecasts, land cover, socio-economic and other datasets. The data is already preprocessed and in a ready-to-use form that removes many barriers associated with data and infrastructure management; this allows users to spend less time downloading data and managing infrastructure and more time on data analysis. Additionally, users can also upload and use their own private data, in conjunction with what is available.
An example of what can be found on the platform is the Landsat data. The Landsat program refers to a series of satellite missions that are managed by both the US Geological Survey and NASA. These satellites are able to track land use efficiently and can indicate land change resulting from climate change, urbanization, and other natural and human factors since 1972.
Another remarkable set of available data refers to Sentinel missions, a constellation of satellites developed by the European Space Agency to operationalize the Copernicus Earth Observation Program. Sentinel-1A and 1B provide all-weather radar images, Sentinel-2A and 2B high-resolution optical images, Sentinel-3 ocean and land data suitable for environmental and climate monitoring.
There are plenty of other available data sets. To name a few:
- Land and sea surface temperature products derived from several spacecraft sensors
- Long-term climate predictions derived from climate models
- Weather datasets describing forecasted and measured conditions over short periods of time, including precipitation, temperature, humidity, and wind, and other variables
- Digital elevation models describing the shape of Earth’s terrain
- Land cover maps describing the physical landscape in terms of land cover classes such as forest, grassland, and water
- Night-time satellite imagery
- Maps of vegetation indices
Understanding what information in the Earth Engine catalog is required to perform your analysis is crucial. Note that the characteristics of these public data sets vary significantly. Some data is being collected on almost a daily basis, such as atmosphere quality information from Sentinel-5P. Other datasets offer a “snapshot” at a specific time, such as Gridded Population Density of the World, which is updated every 5 years.
There are data sets, like from the Landsat missions, that span decades to the past. There are also image collections from newer image providers that can’t offer these time-series analysis capabilities.
Some datasets cover the whole globe but are limited to a coarse spatial resolution, while others cover specific countries or even smaller areas but provide finer details. For example, one pixel of a 180-km-wide Landsat image corresponds to a ground square with a 30m side; on the other hand, one pixel of a 2330-km-wide Terra/Acqua MODIS image corresponds to a ground square with a 500m side.
Check an example of timelapse from Google Earth Engine below
Compute
To handle big data Earth Engine uses the well-known MapReduce paradigm. Introduced by Google in 2004, MapReduce tackles computation on massive volumes of information leveraging the power of parallelization in the most compute-efficient and data-efficient way, and has since then become widely used.
Computation of geospatial images involves several steps, including filtering the pixels of the right time and place, applying data transformations and aggregations, and rendering the results on a map. Such operations must be done on a massive number of pixels and across many different scales and image bands, making MapReduce the perfect solution for this type of problem.
By leveraging MapReduce, Earth Engine allows remote sensing researchers, data analysts and developers to tackle problems that used to take ages (e.g. time-series analysis), in a time-efficient and cost-efficient manner.
New commercial availability
Earth Engine recently became a Google Cloud product and it is now commercially available, allowing its integration into various Google Cloud architectures. Moreover, lots of commercial earth observation satellites, e.g. those owned by Maxar and Planet, are being launched with even more sophisticated sensors for more precise mapping than with publicly available geospatial imagery. Earth Engine is one platform that is able to ingest, store, and process this data, meaning businesses only need to focus on geospatial data analytics and data science.
Geospatial analytics have been proved to be essential for sustainable applications to the ecological, geological, physical, hydrological, and environmental fields. By using Earth Engine, businesses are able to identify and manage their risks to various elements of the planet. For example, these elements include wildfires, changes in the environment, crop health, floods, water sources, and droughts. This can contribute to sustainability in commercial environments and can help tackle climate issues like deforestation, water management, and land use. GEE has made it possible for commercial entities to monitor millions of acres of the earth’s surface in near real-time at scale, without having to struggle with the related infrastructure management and computing challenges.
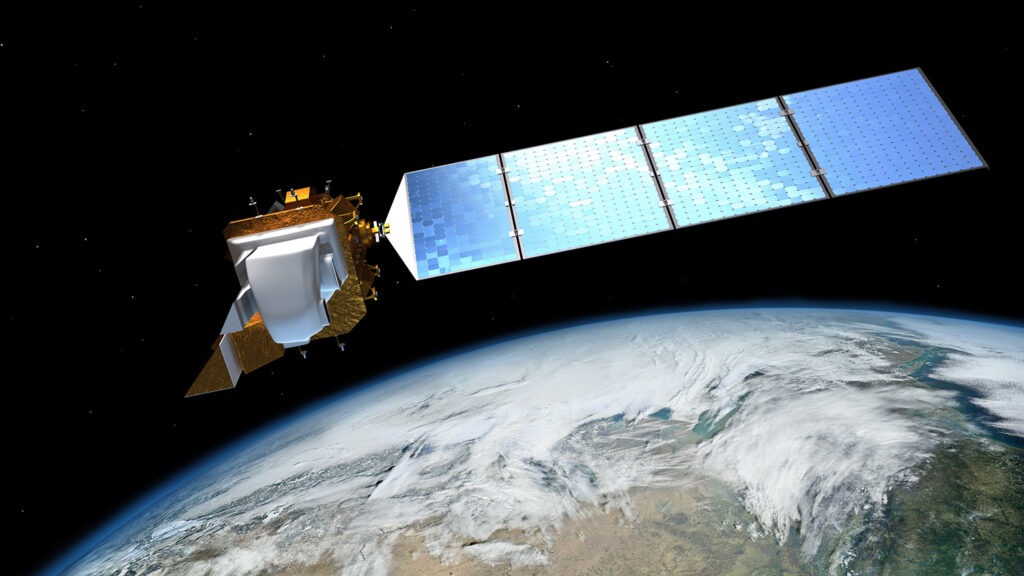
Google Earth Engine use cases
Research and the public sector
GEE was originally launched for scientists and NGOs in 2010 by Google. Every year, more and more papers are being published where researchers tackle urgent global and local problems.
One of the most interesting and recent use cases involves forest conservation in Indigenous territories and protected areas in the Brazilian Amazon in 2023. Here, authors analyzed time-series satellite images and qualified annual forest area in the Brazilian Legal Amazon area under different governance and management regimes. They revealed the vital role of, and substantial progress achieved by, Indigenous territories and protected areas in Amazonian forest conservation as well as the dangers of recent weakening of Brazil’s forest policies.
There are many other case studies to explore how this tool is helping governments and researchers in different strategies and studies involving data and the environment: you can find a list with examples below.
- 2016 High-resolution mapping of global surface water and its long-term changes by Google and the Joint Research Centre of the European Commission.
- 2021 Benefit of woodland and other natural environments for adolescents’ cognition and mental health.
- The U.S. Forest Service uses Earth Engine and Google Cloud to study the effects of climate change, forest fires, insects and disease.
- The Canadian government is also using GEE to map the status and trends of vegetation in the country.
- The Food and Agriculture Organization (FAO) of the United Nations, created Collect Earth, a free, open source tool using Google Earth and GEE to visualize and deforestation in plots of lands.
First commercial application: the Unilever use case
SDGs apply to the private sector as well, as many companies are trying to increase their sustainability and improve their environmental footprint. Earth Engine found its perfect application with Unilever, for monitoring the impact of palm oil harvest on deforestation.
Unilever is one brand that has adopted Google Earth Engine to speed up the restoration of the environment. It aims at ending deforestation, protecting global biodiversity, and limiting climate change. The company announced the formation of a Forest Data Partnership at COP26. This project will also involve the United States Agency for International Development (USAID), Google, US National Aeronautics and Space Administration (NASA), World Resources Institute (WRI), and the UN Food and Agriculture Organization (FAO).
This coalition is meant to create a unified framework that will let everyone in the world monitor how much progress we are making in reducing deforestation and restoring degraded land. It will focus on several key places. These include the Amazon Basin, West Africa, and South-East Asia. The coalition aims at bringing in more committed companies, governments, and NGOs.
The role of Accenture
Businesses are already using Google Cloud for sustainability through Earth Engine. Accenture, a Fortune 500 Global company, has partnered with Google Cloud to help its clients gain the full benefits of the cloud and data on their path to more sustainable operations. It encourages companies to use cloud technology to tackle food waste, create low-carbon transport, cut waste in customer service, and adopt other sustainable practices. You can learn more here about how this partnership is combining technology, data, and sustainable cloud practices to bring companies closer to global sustainability goals.
Accenture is aware of the strategic importance of earth observation, and has in fact already established partnerships with satellite imagery providers and geospatial system integrators , and is already helping many clients across different industries (fashion, consumer packaged goods, telecommunications, and others) to use tools such as GEE to increase visibility over their processes. Moreover, Accenture has been awarded the 2022 Terra Carta Seal, which recognizes global companies that are driving innovation and demonstrating their commitment to, and momentum towards, the creation of genuinely sustainable markets.
How devs and data analysts can use GEE
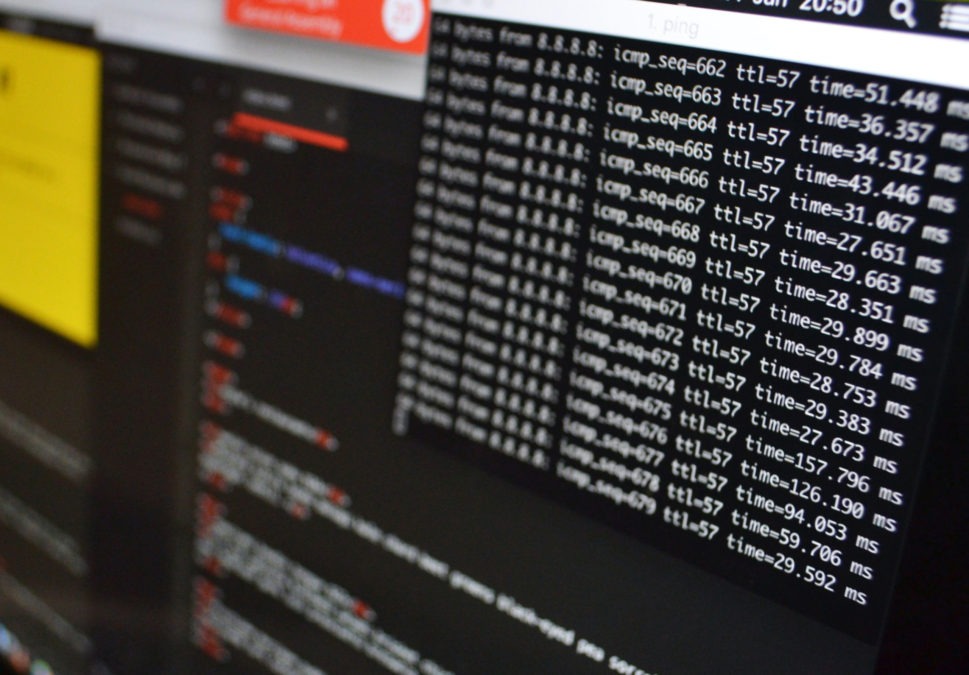
Google Earth Engine has several options that allow you to analyze data. They include:
- Online Code Editor– This first option is to use the online code editor in the GEE interactive environment. It is ideal for prototyping and iteration. The center panel of this tool provides a JavaScript code editor equipped with a map and a console for outputting the results. Users appreciate the speed it provides because no software or data need to be downloaded locally.
- Using Python notebooks– GEE also works in Python notebooks like Colab. This particular product was developed by Google Research and allows anybody to write python code through their browser and share it via Google Drive, all while using the very popular programming language across data scientists i.e. Python. It is thus specially developed for data analysis and machine learning.
- Earth Engine apps- Dynamic, publicly accessible user interfaces for Earth Engine analyses. Scripts created in the online Code Editor can be easily published as interactive web apps with graphical interface. There is no need to run any configurations or manage your servers as everything is handled by Google. Many researchers choose this option to share results of their study.
Earth Engine also offers great integrations with other services on Google Cloud Platform, such as BigQuery for integration with other data sources, Cloud Run and Coud Functions for PaaS event-driven pipelines, and Vertex AI for deriving new insights through the application of machine learning algorithms to highly unstructured data as satellite imagery.
Conclusions: The future of big data analysis for sustainability?
Advancements in big data analysis will make it possible for companies and governments to anticipate problems resulting from climate change thanks to forecasting algorithms leveraging AI. This will help with more accurate planning. The technology will also make it possible for systems to present data in an engaging way. This will create more interest in dealing with climate change and the depletion of planet resources, and that will make the general public more interested in sustainability.
Big data analysis will also offer more and more opportunities for transparency. Scientists will focus more on analytics engineering and will aim at modeling data in a way that empowers end users to answer their own questions. This way, we will switch from a certification mentality to direct verification.
To improve data transparency, scientists will also focus on developing no-code solutions. These will make it easier for stakeholders to understand and evaluate the data without pestering specialists.
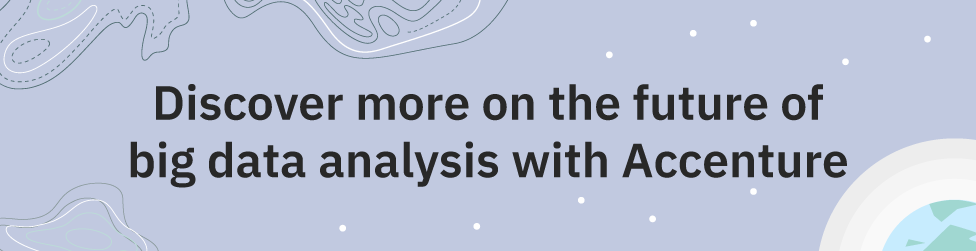
This article was written with the collaboration of Accenture's Dario Balestri, ML Engineering Associate Manager, and Yaroslav Vasyunin, ML Engineering Senior Analyst.