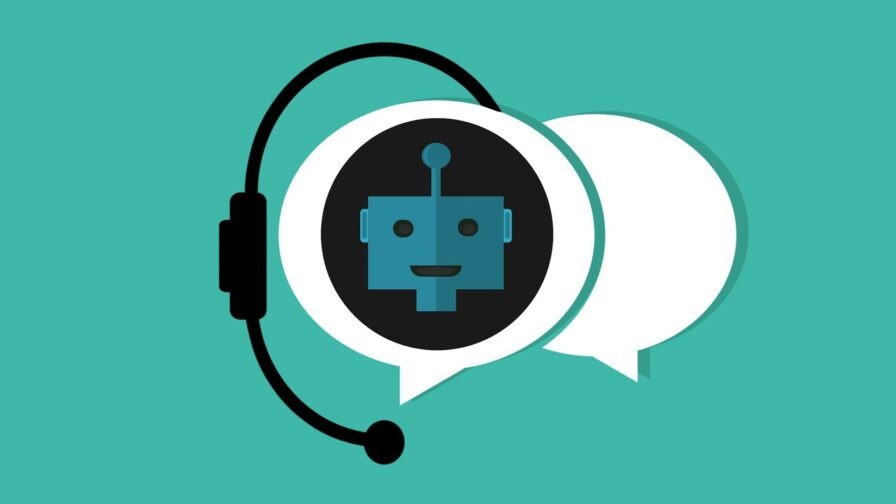
“Create an image of what you think I look like based on what you know about me.”
The internet is flooded with AI-generated portraits—some cartoon-like, some flesh-made—of people’s appearances, often accompanied by comments on how little resemblance these pictures bear to their original subjects.
My output was a not-so-good-looking male, which made me raise an eyebrow. How can ChatGPT not do a better job with all the information I provided? It should know I’m a woman, at the very least.
Scrolling through LinkedIn, I stumbled upon a fellow female sharing the same sentiment. LLMs are biased toward male input and, therefore, are more likely to assume you’re male by default.
It made me question. The technology that is being pitched as revolutionary, intuitive, and borderline human still leans into a worldview that is stubbornly unbalanced.
The gender biases of Large Language Models (among others)
Bias in AI isn’t new, but it takes on new shades when it shows up in tools millions of people interact with daily. The training data behind LLMs is vast and indiscriminate—scraped from blogs, forums, news sites, Reddit threads, Wikipedia pages, and more. Unsurprisingly, this means the biases baked into our digital spaces are inherited and amplified by these models.
Statistically, male perspectives dominate the online space. From the way questions are phrased to the examples provided, the model is simply reflecting its environment. That’s not an excuse—it’s the root of the problem. If LLMs are being designed to assist, inform, and interact with humans of all kinds, their assumptions shouldn’t be shaped so strongly by one demographic’s voice.
Research supports this observation. A study by University College London found clear evidence of bias against women in content generated by several LLMs. Female names were strongly associated with words such as “family,” “children,” and “husband,” conforming to traditional gender roles. In contrast, male names were more likely linked to terms like “career,” “executives,” “management,” and “business”.
When technology that’s meant to serve a global population defaults to calling women “he,” or assumes doctors are men and nurses are women, we’re not dealing with a futuristic tool. We’re just seeing old patterns dressed up in new code.
Misinformation dressed as authority
Then comes the issue of authority. Because LLMs communicate in clean, confident prose, their outputs are often taken at face value—even when they’re dangerously wrong.
People turn to these tools for medical advice, legal information, and educational content. But unlike a professional with credentials, an LLM doesn’t “know” anything. It patterns its answers from the statistical likelihood of certain word combinations appearing together.
This makes them incredibly useful for drafting emails or brainstorming, but dangerously misleading when users assume factual correctness. When the tone is persuasive, but the content is flawed, who carries the responsibility for the fallout?
Consider the case of Arve Hjalmar Holmen, a Norwegian man who filed a complaint against OpenAI after ChatGPT falsely claimed he had murdered his two sons. ChatGPT’s fabricated response not only defamed Holmen but also highlighted the potential for AI to disseminate harmful misinformation.
Similarly, during the 2024 U.S. elections, ChatGPT provided incorrect answers to questions about voting procedures in battleground states, raising concerns about the spread of misinformation in critical democratic processes.
Even seemingly harmless errors can have a compounding effect. For example, in academic settings, students using LLMs to research or summarize topics may unknowingly build essays on flawed foundations. When citations are invented, facts misrepresented, or nuance lost, the resulting output isn’t just inaccurate; it’s misleading in a way that feels trustworthy.
The polished tone is part of the issue. It encourages trust where caution is needed. The more these tools are deployed in consumer-facing contexts, the more urgent it becomes to treat their content not as authoritative, but as drafts or suggestions, not truths.
Privacy concerns: Your data isn’t as private as you think
Conversations with LLMs can feel oddly intimate, but let’s not forget: interacting with these tools means feeding them your data. And while companies claim your inputs aren’t stored—or are anonymized—what “anonymized” means in practice is often vague and difficult to audit.
There are gray zones, especially in workplace applications or customer support tools powered by AI. If you type in sensitive personal or financial information, where does it go? Who sees it? Can it resurface later?
The line between user input and training data is blurry. And it should make us all a bit uncomfortable.
A blog post by Sentra highlights that LLMs can be configured to allow users to provide inputs that may contain sensitive information. If these inputs are not adequately filtered or sanitized, they can be incorporated into the LLM’s training data, potentially leading to the disclosure of sensitive information.
Moreover, discussions on platforms like Reddit reveal growing concerns about privacy when using AI models. Users worry that everything inputted into an LLM might be stored and potentially exposed during data leaks or subpoenas.
And as governments and regulatory bodies struggle to catch up, users are left navigating a minefield of terms and conditions that few truly understand. It raises the question: should we really have to think twice before asking a virtual assistant a personal question?
So where do we go from here?
We can’t uninvent LLMs. They’re here, and they’re only getting more embedded into the tools we use and the systems that govern our daily lives. The question is not whether we move forward, but how.
Some potential steps we can take to create better technology:
- Transparent training practices: We need visibility into the data sources used and more critical curation, not just bulk scraping.
- Bias auditing: Models should undergo continuous, third-party audits for gender, racial, and cultural bias, and developers should be held accountable for acting on those findings.
- User control: Let people opt out of having their data used for training and provide clearer boundaries for what’s stored, when, and for how long.
- Stronger disclaimers: Don’t let the tone of confidence fool users. AI-generated content should come with built-in caveats.
- Ethics by design – Integrate ethical questions into development cycles, not as afterthoughts or PR responses.
At the heart of it, LLMs are not neutral tools. They reflect the values, priorities, and oversights of the societies that built them. As we integrate these systems deeper into our lives, we owe it to ourselves, and to each other, to question what they amplify, what they erase, and who gets to shape their voice.
The responsibility doesn’t lie solely with engineers or ethicists; it belongs to all of us who choose to engage, critique, and demand better. Only through that collective scrutiny can we hope to shape a future where AI serves everyone. Fairly, transparently, and responsibly.