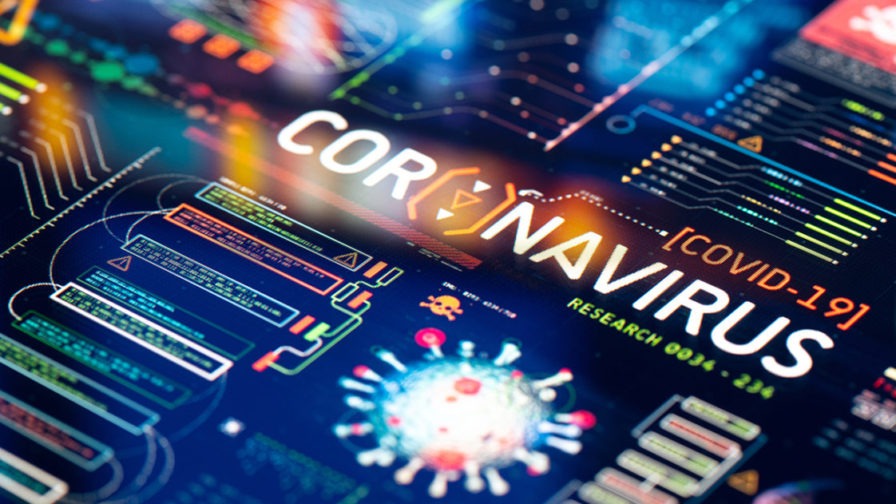
The SARS-CoV-2 coronavirus and the associated COVID-19 disease have totally dominated our lives this year. The virus has brought death and hardship on a truly biblical scale. The fight against the virus has sparked a huge research effort. In the run up to the Codemotion conference on deep learning, we look at how researchers have turned to machine learning and artificial intelligence for help. These include using unsupervised learning to find potential drug therapies and using ML to determine when to relax quarantine.
What is COVID-19
COVID-19, or coronavirus disease 2019, is the official name for the acute respiratory disease caused by the SARS-CoV-2 coronavirus. COVID-19 is characterised by a high fever and a persistent dry cough. As it develops further, it leads to severe pneumonia and, ultimately, respiratory collapse. Currently, there are no approved treatments or vaccines for COVID-19. As a result, the only way to contain the disease is through testing, contact tracing and isolation.
The challenge of testing
Testing for SARS-CoV-2 is challenging. Most tests rely on a process called reverse transcription polymerase reaction or RT-PCR. This test works by finding traces of the virus’s RNA within a sample. The test is usually very accurate, although there are reports of up to 30% false negatives (see later for why this matters). The real problem is, this test is slow, requires specialised machines and needs chemicals that are in short supply. These constraints mean no large country has managed to test more than ~2.5% of their population.
Another approach relies on serology. That is, it looks for the presence of antibodies within a patient’s blood. If there are antibodies present, that means the patient has previously been infected with the coronavirus. As things stand, no antibody test has been proven to be effective enough for use in the field. And even if someone has antibodies, it won’t prove they are either immune or no longer carriers of the virus.
False positives and false negatives
Any test will always give a number of false results. False positives will incorrectly label someone as suffering from COVID-19. This can be a problem if they subsequently recover and believe they are now immune. To try and reduce this risk, most patients are tested multiple times. The bigger problem is with false negatives. That is tests that incorrectly say someone is free from SARS-CoV-2 when they aren’t. This is a much more significant problem because it means people that are infectious believe that they aren’t. This is one key reason why so many countries insist on quarantine for anyone with COVID-19 symptoms.
Turning to machine learning
In a previous article, we already described the risks of developers and computer scientists dealing with viruses and the available epidemic data. Nonetheless, here we try to recap the most significant current efforts to create machine learning models for diagnosing people with COVID-19.
Researchers in two labs have turned to an alternative approach for testing. They are trying to establish if you can detect patients with COVID-19 from how they sound. In both cases, the researchers are using machine learning for this. However, their methodology is slightly different. Professor Cecilia Mascolo of the University of Cambridge Computer Laboratory is leading one effort. The other team is led by Dr Rita Singh at Carnegie-Mellon University and builds on her earlier work on voice profiling.
The COVID-19 Sounds Project at Cambridge is currently collecting data from as many people as possible. They are doing this using an app, which is available on the Google Play Store, online, and (soon) on iOS. The app asks you to record yourself reading aloud, coughing and breathing. You provide basic demographics (e.g. age, gender). You are also asked to self-report whether you are suffering from COVID-19 or not. Importantly, the app is anonymous—it collects no direct identifiers. This data will be used to train machine learning models that seek to predict whether someone has COVID-19.
The team at Carnegie-Mellon are working on a COVID Voice Detector. As already mentioned, their system builds on their existing voice-profiling models. As with the Cambridge project, their system needs users to provide recordings of their voice. In this case, you are asked to cough several times, recite the alphabet, and then record a set of vowel sounds. The initial version immediately gave you a score indicating how likely it thought you were to have COVID-19. However, within a very short time, the team realised this was a potential problem since there was no evidence for how accurate the test was. As Dr Singh said in a BBC report:
“If a system tells a person who has contracted COVID-19 that they don’t have it, it may kill that person. And if it tells a healthy person they have it, and they go off to be tested, they may use up precious resources that are limited. So, we have very little room for error either way and are deliberating on how to present the results so that these risks vanish.”
Other teams are working on similar approaches. For instance, a team the University of Washington is working on a system to identify and classify coughs.
How will ML diagnosis work?
Both the projects above are hoping that they can identify a unique “fingerprint” for how COVID-19 affects how we sound. In particular, they hope that there will be distinct differences compared to other illnesses and diseases that affect the respiratory tract. As the team at Carnegie-Mellon say on their website:
“The sound of our voice (regardless of language), and the sounds we make when we breathe or cough change when our respiratory system is affected. The changes range from coarse, clearly audible changes, to minute changes — what we call “micro” signatures, that are not audible to the untrained listener, but are nevertheless present.”
At present, it isn’t known whether this is actually possible, nor whether the resulting model will be accurate. However, last year, a team at Curtin University and The University of Queensland, Australia reported that they had developed an app to diagnose respiratory diseases using a similar approach. If this approach does work for COVID-19, it can potentially be used to speed up initial diagnosis over the phone. This will allow more accurate targeting of scarce medical resources. Even if it isn’t completely successful, the projects are expected to create huge datasets. These should prove useful for other studies into the physiological effects of COVID-19.